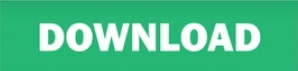
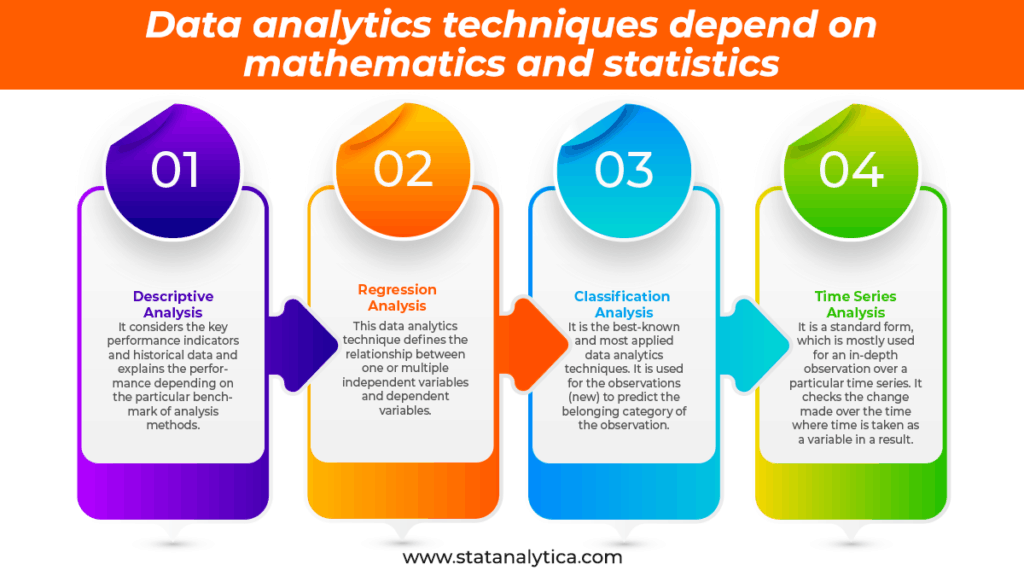
- #Basic data analytics methods using r archive
- #Basic data analytics methods using r software
- #Basic data analytics methods using r code
- #Basic data analytics methods using r plus
- #Basic data analytics methods using r free
Stefano Iacus, Guido Masarotto, Heiner Schwarte, Seth Falcon, Martin Morgan, and Duncan Murdoch were members.
#Basic data analytics methods using r plus
As of January 2022, it consists of Chambers, Gentleman, Ihaka, and Mächler, plus statisticians Douglas Bates, Peter Dalgaard, Kurt Hornik, Michael Lawrence, Friedrich Leisch, Uwe Ligges, Thomas Lumley, Sebastian Meyer, Paul Murrell, Martyn Plummer, Brian Ripley, Deepayan Sarkar, Duncan Temple Lang, Luke Tierney, and Simon Urbanek, as well as computer scientist Tomas Kalibera. The R Core Team was formed in 1997 to further develop the language. As of December 2022, it has 103 mirrors and 18,976 contributed packages. CRAN originally had three mirrors and 12 contributed packages.
#Basic data analytics methods using r archive
Its name and scope mimics the Comprehensive TeX Archive Network and the Comprehensive Perl Archive Network. The Comprehensive R Archive Network (CRAN) was founded in 1997 by Kurt Hornik and Fritz Leisch to host R's source code, executable files, documentation, and user-created packages. The first official 1.0 version was released on 29 February 2000. R officially became a GNU project on 5 December 1997 when version 0.60 released. Mailing lists for the R project began on 1 April 1997 preceding the release of version 0.50.
#Basic data analytics methods using r free
In June 1995, statistician Martin Mächler convinced Ihaka and Gentleman to make R free and open-source under the GNU General Public License. Ihaka and Gentleman first shared binaries of R on the data archive StatLib and the s-news mailing list in August 1993. The name of the language comes from being an S language successor and the shared first letter of the authors, Ross and Robert. The language took heavy inspiration from the S programming language with most S programs able to run unaltered in R as well as from Scheme's lexical scoping allowing for local variables. R was started by professors Ross Ihaka and Robert Gentleman as a programming language to teach introductory statistics at the University of Auckland. Multiple third-party graphical user interfaces are also available, such as RStudio, an integrated development environment, and Jupyter, a notebook interface. Precompiled executables are provided for various operating systems. It is written primarily in C, Fortran, and R itself (partially self-hosting).
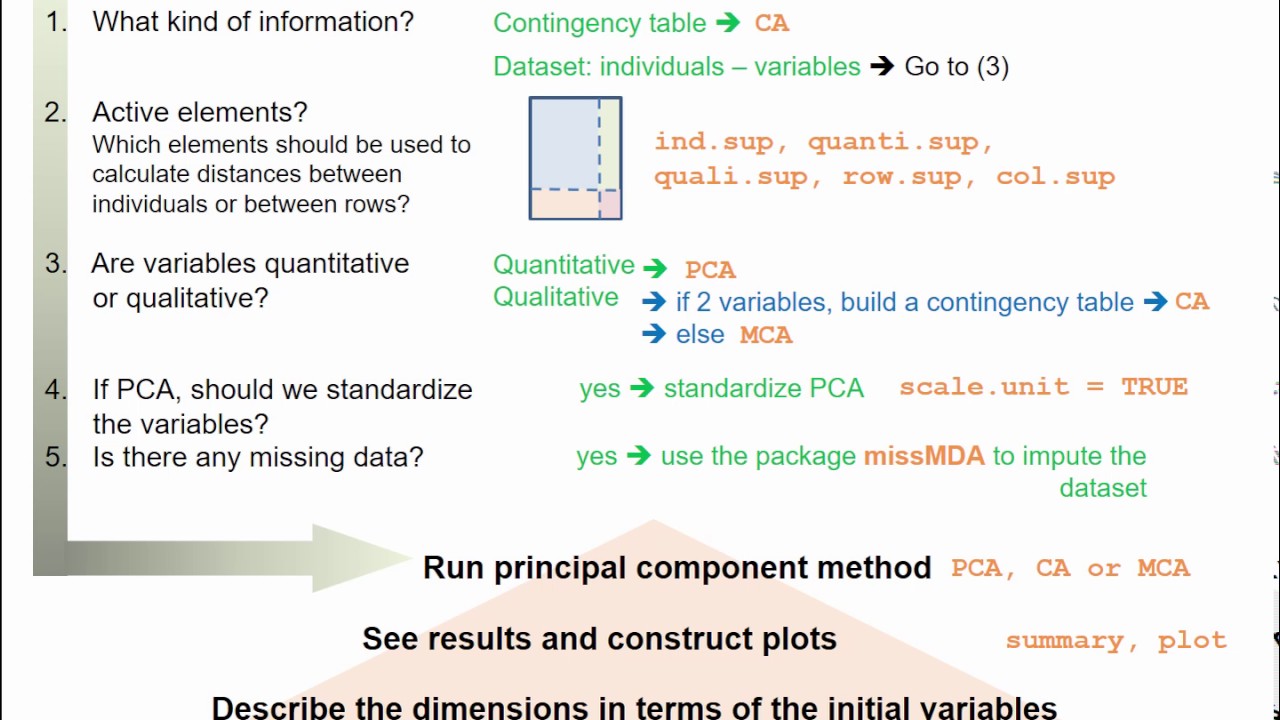
#Basic data analytics methods using r software
The official R software environment is an open-source free software environment released as part of the GNU Project and available under the GNU General Public License. As of April 2023, R ranks 16th in the TIOBE index, a measure of programming language popularity, in which the language peaked in 8th place in August 2020.
#Basic data analytics methods using r code
The core R language is augmented by a large number of extension packages containing reusable code and documentation.Īccording to user surveys and studies of scholarly literature databases, R is one of the most commonly used programming languages in data mining. Created by statisticians Ross Ihaka and Robert Gentleman, R is used among data miners, bioinformaticians and statisticians for data analysis and developing statistical software. Instructors, contact your Pearson representative for more information.R is a programming language for statistical computing and graphics supported by the R Core Team and the R Foundation for Statistical Computing. Students, if interested in purchasing this title with MyLab Economics, ask your instructor to confirm the correct package ISBN and Course ID. Note: You are purchasing a standalone product MyLab Economics does not come packaged with this content. Also available with MyLab Economics By combining trusted author content with digital tools and a flexible platform, MyLab(tm) personalizes the learning experience and improves results for each student. This coverage and approach make the subject come alive for students and helps them to become sophisticated consumers of econometrics. With very large data sets increasingly being used in economics and related fields, a new chapter dedicated to Big Data helps students learn about this growing and exciting area. The text incorporates real-world questions and data, and methods that are immediately relevant to the applications. The 4th Edition maintains a focus on currency, while building on the philosophy that applications should drive the theory, not the other way around. Engaging applications bring the theory and practice of modern econometrics to life Ensure students grasp the relevance of econometrics with Introduction to Econometrics - the text that connects modern theory and practice with motivating, engaging applications. For courses in introductory econometrics.
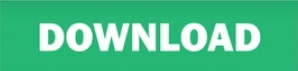